Convolutional Neural Networks with Transfer Learning for Pneumonia Detection
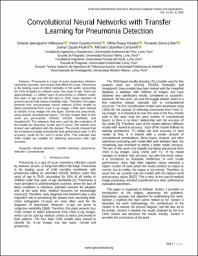
Ver/
Descargar
(application/pdf: 854.1Kb)
(application/pdf: 854.1Kb)
Fecha
2022Autor(es)
Iparraguirre-Villanueva, Orlando
Guevara-Ponce, Victor
Roque Paredes, Ofelia
Sierra-Liñan, Fernando
Zapata-Paulini, Joselyn
Cabanillas-Carbonell, Michael
Metadatos
Mostrar el registro completo del ítemResumen
"Pneumonia is a type of acute respiratory infection
caused by microbes, and viruses that affect the lungs. Pneumonia
is the leading cause of infant mortality in the world, accounting
for 81% of deaths in children under five years of age. There are
approximately 1.2 million cases of pneumonia in children under
five years of age and 180 000 died in 2016. Early detection of
pneumonia can help reduce mortality rates. Therefore, this paper
presents four convolutional neural network (CNN) models to
detect pneumonia from chest X-ray images. CNNs were trained
to classify X-ray images into two types: normal and pneumonia,
using several convolutional layers. The four models used in this
work are pre-trained: VGG16, VGG19, ResNet50, and
InceptionV3. The measures that were used for the evaluation of
the results are Accuracy, recall, and F1-Score. The models were
trained and validated with the dataset. The results showed that
the Inceptionv3 model achieved the best performance with 72.9%
accuracy, recall 93.7%, and F1-Score 82%. This indicates that
CNN models are suitable for detecting pneumonia with high
accuracy."
Colecciones
- SCOPUS [381]